Big data analytics is now key to driving artificial intelligence efficiency in business.
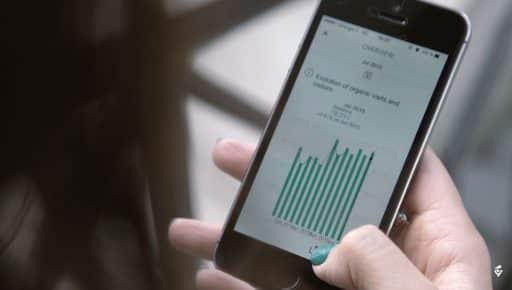
© Captain Dash
Deep learning, machine learning, neural networks… artificial intelligence (AI) jargon was everywhere in 2016 and remains so in 2017. “The potential of AI seems to be significant,” note Gilles Babinet and Bruno Walther, co-founders of Captain Dash, a company that provides decision support solutions in the form of dashboards (1). “Whoever has had the opportunity to test chatbots in, say, a stock management or management control context is bound to have been impressed by the speed of understanding demonstrated by these new tools, which increasingly feature natural language interfaces.”
But it’s important to be able to analyse the lessons learned from AI without overlooking certain key points and taking the risk of misinterpreting them. In the US, for example, machine learning data intended for police forces resulted in identity checks that discriminated against some ethnic minorities.
To avoid this kind of situation, using big data analytics is the advisable course of action. “This is where analytics comes into its own: by enabling complex reports to be visualised in a concise manner, faults can be flagged up that haven’t been identified by machines. An experienced operator can then correct the faults and, in doing so, educate the machine,” add Gilles and Bruno.
Team of 60 people at Axians Netherlands
Such issues are at the core of operations at Axians Netherlands (VINCI Energies), where a team of 60 people works on AI and big data in the healthcare, industrial, and retail sectors. “If you want to apply artificial intelligence properly, you first need to have real experience in these markets and understand the problems and opportunities they face,” says Edwin Van Merriënboer, business unit manager.
“We developed a predictive model populated with lots of data which improved sales predictions by 10 to 20%.”
For example, in industry one of the major challenges is maintenance – a very expensive cost item for companies. “Many engineers have valuable maintenance expertise, but when they retire, the information disappears. Inserting this knowledge of maintenance processes into AI-based solutions ensures that processes continue to run effectively once the engineers have gone,” he explains.
To take another example: in a flower retail business an experienced sales representative from a company would predict the quantity of flowers expected to be sold in coming weeks. “The challenge we set ourselves for the client was to produce an algorithm that would make better predictions. We developed a predictive model populated with lots of data – sales history, weather data, and so on – which improved sales predictions by 10 to 20%. The client was very happy. And the sales rep was able to focus on core sales duties. AI algorithms aren’t there to replace staff but to help them use their talents more effectively,” states Edwin.
17/10/2017